Oklahoma State University CAS faculty conduct research using AI machine learning technologies
Tuesday, September 12, 2023
Media Contact: Elizabeth Gosney | CAS Marketing and Communications Manager | 405-744-7497 | egosney@okstate.edu
Artificial intelligence (AI) has become a hot topic in recent months because of its ability to rapidly perform various complex tasks. But, it has caught a negative connotation in academia due to its use by students.
However, researchers in Oklahoma State University’s College of Arts and Science use a subfield of AI — machine learning — for positively impactful research studying complex data sets.
Detecting Disease
Dr. Lucas Stolerman, assistant professor in the Department of Mathematics, conducted research using digital traces to build prospective and real-time county-level early warning systems using machine learning to anticipate COVID-19 outbreaks in the United States.
During his postdoctoral year at the Boston Children’s Hospital and Harvard Medical School, Stolerman initially began conducting this research under Dr. Mauricio Santillana and in collaboration with Leonardo Clemente a doctoral student.
During his first year on faculty at OSU, the project was finalized.
“Our goal was to detect potential COVID-19 outbreaks in U.S. counties ahead of time,” Stolerman said. “To this end, we created a machine learningbased early warning system leveraging internet-based digital data.”
The early warning system provides an alert when the digital data increases up to a certain level. The digital datasets included Twitter microblogs and Google trends terms including “Covid,” “Covid19,” “How Long Does Covid Last,” “Covid Symptoms,” “Fever,” “Cough” and others.
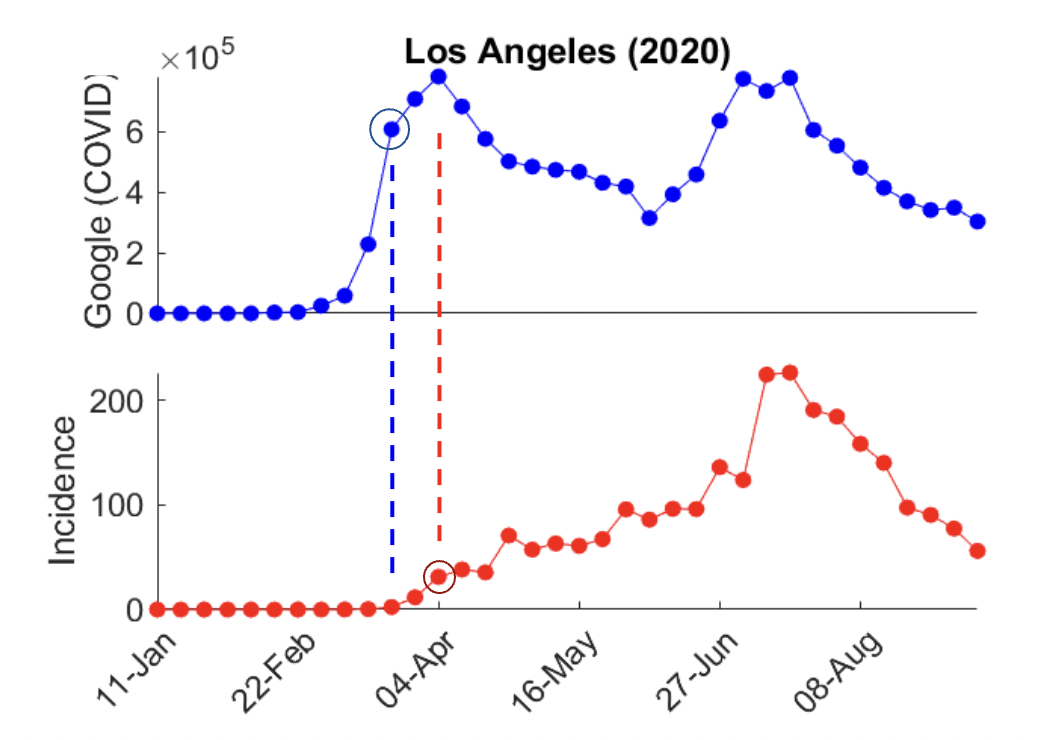
By training the models over historical data, the researchers could then perform out-of-sample predictions and assess their method’s ability to correctly predict COVID-19 outbreaks.
“COVID-19 outbreaks result from a complex combination of multiple factors, including viral mutations, social interactions, vaccination and human behavior. A powerful message from our study is that digital traces can help detect when those outbreaks will take place in U.S. counties,” Stolerman said.
This research resulted in machine learning methods that leverage internet-based digital traces to anticipate sharp increases in COVID-19 activity in U.S. counties.
“Whether using artificial intelligence to investigate and predict the spread of diseases or adapting advanced computational techniques to tackle relevant questions in biology, Dr. Stolerman’s research shows the wide applicability of mathematics and its deep connections with other sciences,” said Dr. Jay Schwieg, head of the Department of Mathematics.
Pondering the Past
Dr. Howard Sanborn, professor and political science department head, received a grant through the U.S. Consulate in Hong Kong to collect legislative data and use machine learning models to assess government responses to crises in Hong Kong.
While investigating legislative politics in Hong Kong, the 2019 Core Fulbright Scholar noticed a series of changes in how the city was managed based on the action and reaction to conflicts.
China and most of the former and current British Commonwealth countries have comprehensive online databases in English, Chinese and Cantonese of their government documents, particularly legislative proceedings. This allows Sanborn and his team to study protests at discrete points in Hong Kong history, reaching as far back as the 1850s.
The goal is to see what words and phrases appear in conversations frequently before, during and after these conflicts. However, combing through the database for government debates and deliberations could take years.
“I applied for the grant to be able to deploy machine learning computing techniques to process a lot of documents all at once with the driving goal of trying to understand what the government responses are to crises,” Sanborn said.
The initial grant assists with the hiring of students experienced in machine learning and political science.
“Right now, we’re starting more with an unsupervised model where we’re picking out just a small period and looking at the government debates and essentially refining the modeling,” Sanborn said.
Together, a statistics graduate student works on machine programming while a political science graduate student helps identify the context of the phrases for each date and session to see what generates from the unsupervised model. As the process continues the model refines.
“Transdisciplinary research is a big deal. And I believe in it. ... I think our math, computer science and statistics departments really have a lot to offer in particular to the social sciences, and so I’m hoping this is a step towards that,” Sanborn said.
Studying Stress
The U.S. has the world’s largest fed-cattle industry and is the world’s largest consumer of beef.
In Oklahoma, the beef industry represents a significant agricultural commodity. However, mortality from stress in feedlot cattle threatens the sustainability of the industry and is intensified by extreme weather conditions and trends to select animals for high growth rates.
No resources are currently available for producers to identify animals prone to stress and related mortality. Researchers in CAS and the Department of Animal and Food Science were awarded a United States Department of Agriculture (USDA) Agriculture and Food Research Initiative (AFRI) grant program of the National Institute of Food and Agriculture: Inter-Disciplinary Engagement in Animal Systems (IDEAS) to develop tools that help identify these animals.
“The purpose of this research is to develop precision livestock management tools that use artificial intelligence and machine learning to identify cattle that are susceptible to stress,” said Ashley Railey, sociology assistant professor and project co-principal investigator. “Part of this research, and that which is augmented by sociology, is understanding how to disseminate the research to producers and how the tools can best be implemented amongst producers.”
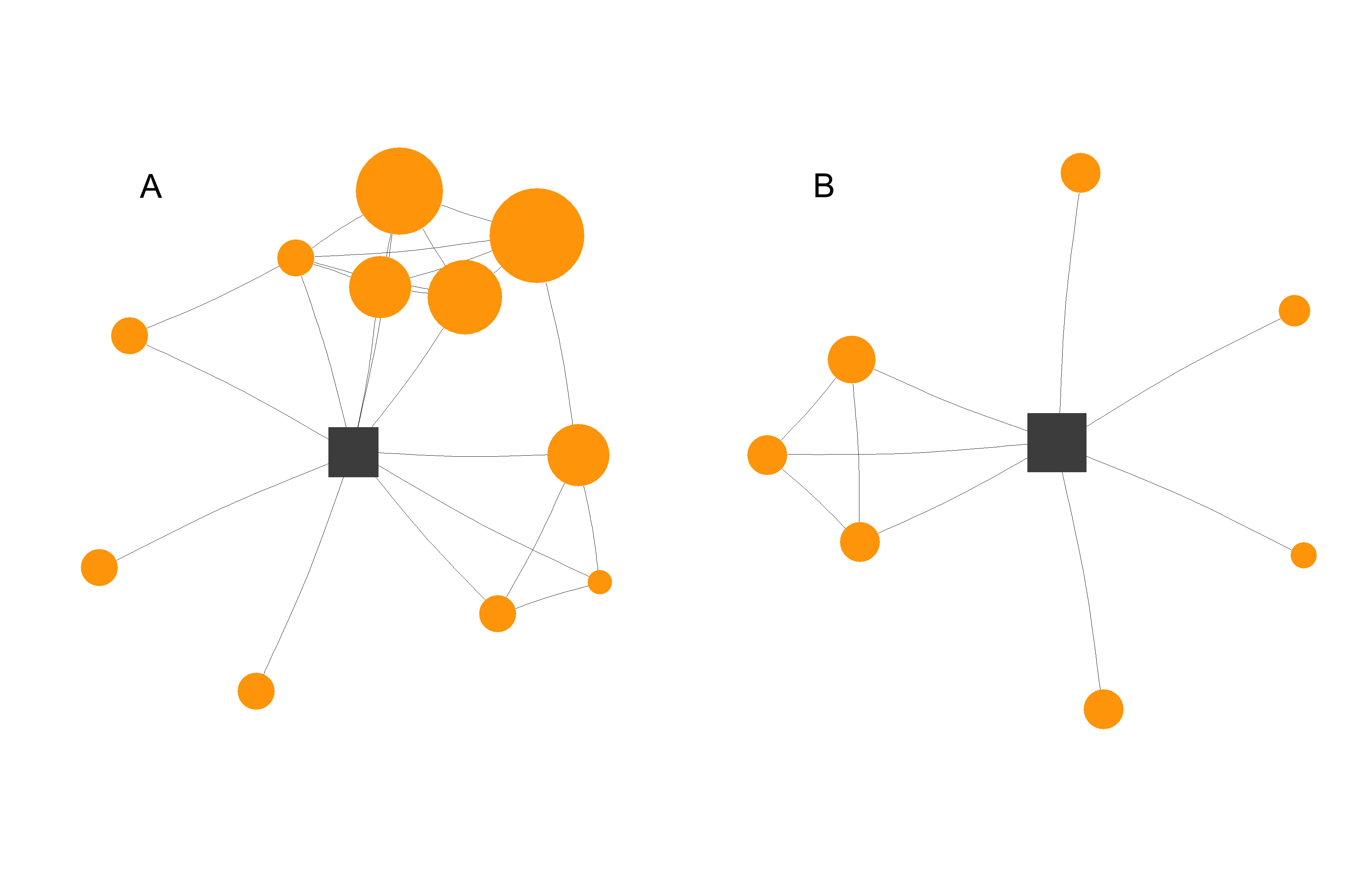
Each co-investigator brings preliminary data to begin the project, with Railey’s initial research suggesting producers value early detection when avoiding major economic impacts, such as mortality of animals. By working with extensions offices, the uncertainty associated with the new technologies can be reduced by allowing producers to evaluate whether the technology is appropriate for their feedlots.
“Artificial intelligence is used to sort through the large amounts of data from tracking animal behavior over time on the farm feedlot,” Railey said. “We will receive data from sensors on the animals and of the climate, video and audio of the farm or feedlot.”
Led by Dr. Ning Wang, biosystems and agricultural engineering professor, and Dr. Sathyanarayanan Aakur, computer science assistant professor, the AI framework will continuously monitor data and derive insights for assessing stress levels by tracking animal behavior over time.
“In the AI field, limited studies have utilized vision,” Railey said. “In this research, Dr. Aakur’s expertise in vision AI — using images to predict an outcome — is very unique.”
Machine Learning in Academia
To discover more about what CAS faculty are doing in the world of AI and machine learning research, listen to a recent episode of the College of Arts and Sciences’ podcast where in Center for the Humanities’ members Dr. Rosemary Avance, Dr. Heather Stewart and Richard Sylvestre discuss effects of ChatGPT and other machine learning bots on academia.
Story By: Sydney Trainor | CONNECT magazine